Why Sentiment Analytics Should Be A No-Brainer For Organisations
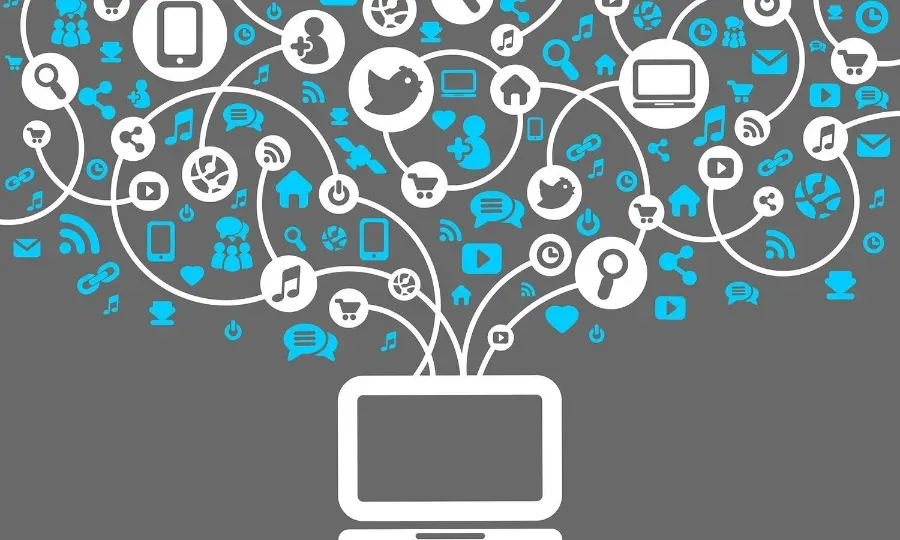
The number of citizens that are active on a social network continues to grow rapidly. At the moment there are more than 1 billion Facebook users, of which around 850 million are active on a monthly basis. There are more than 100 billion connections and each day gives us 2.7 billion new likes. Twitter has 500 million users and 180 million tweets are sent every day. Approximately 100 million Twitter users are active on a monthly basis. LinkedIn has 225 million users and almost 175.000 users sign-up every day. Pinterest has over 10 million users, of which 97% are women. Instagram has over 5 millions images uploaded every day and Google+ receives 5 billion +1’s every day.
These are only the social networks developed in the Western world. There are numerous social network from Asia that you probably have never heard of, but that are as big or bigger as let’s say Twitter. Qzone, a website were users can create blogs, share photos and music and much more has 712 million users. The Chinese edition of Twitter, Tencent Weibo, has 507 million users. Sina Weibo, a hybrid version of Facebook and Twitter, has 500 million users. Wechat, a micro-messaging app similar like WhatsApp, has 300 million users. Social media is huge and drives massive amounts of data on a daily basis, worldwide.
With the rise of the social media networks, came the ability to understand what people say online, why they say it and what their opinion is about products or services. Sentiment analytics is therefore also called opinion mining and it means the application of data analytics to social network data to understand how people think about certain topics. Using social media algorithms it is possible to understand the sentiment of your customers in real-time, knowing how they think about new products, services or commercials. In addition, it can be used to find the most important influencers.
Sentiment analysis uses natural language processing (NLP), text mining and data mining capabilities to find subjective information hidden in the data. This information refers to the attitude of the data, whether this is positive, negative or neutral. Proper sentiment analysis has to take into account the meaning of the words as well as the context when someone said something through what channel.
NLP gives machines the ability to read and understand as well as derive meaning from the languages humans speak, and it is part of Artificial Intelligence. The challenge with NLP lies in the fact that computers normally requires humans to speak the programming language, which has to be unambiguous and highly structured, while natural language is anything but unambiguous.
One of the reasons proper NLP is so difficult is because of the variability of the data. Variability means that the meaning is changing (rapidly). In (almost) the same tweets a word can have a totally different meaning. In order to perform a proper sentiment analyses, algorithms need to be able to understand the context and be able to decipher the exact meaning of a word in that context. This is still very difficult.
The advantages are however ubiquitous. Using a sentiment analysis, companies can understand what their customers think of their service / product offering / latest commercial etc. Even more, all the available social data can be used to perform predictive analysis about what customers want and when they want it. Based on the feedback they post on the social networks, companies can obtain insights that would normally require expensive traditional research. This is done especially in the entertainment industry, where online sentiment analyses are a very good predictor about whether or not a movie will become a success.
In the entertainment industry, sentiment analytics is combined with historical data about different shows such as when a user pauses, forwards, rewinds, replays or stops a TV series provides valuable information. Combined with a very detailed (social) profile of the person watching as well as many different tags related to series or movie created by viewers and there is an extremely valuable data stream that provides insights whether or not series or a movie will be a success. Without proper sentiment analytics, this would not be possible.
Sentiment analytics is valuable for almost any company in any industry. The analysis of what is being said online will provide retailers with additional insights into what customers are really looking for and it will enable retailers to optimize their assortments to the local needs and wishes. Earlier I already covered Nestlé, as they track the sentiment of their 2.000 brands to know what their customers think, to deliver products that they want and to prevent crisis’s from happening. Governments can use big data sentiment analytics to discover potential areas of civil unrest and this can help to take preventive action if required. It can help to address issues before they spread too big and can help to improve the service.
There are many different companies that offer sentiment analytics solutions. These innovative and sophisticated SaaS solutions offer companies the ability to really start to understand your customers. A few of them we reviewed on this platform before. Crimson Hexagon for example can help organisations with information about how customers really feel about a brand, how they react to advertising or what the most important drivers are for customers to buy a product. Another one is Semantria that enables their users to easily monitor various (social media) channels and gain a better understanding of the voice of the customer.
Social sentiment analytics should really be a no-brainer for all organisations that want to better understand their customers and to deliver products that better fit with the needs of those customers. It offers marketers the wisdom of the crowd and gives them a focus group of millions of customers that can be tracked in real-time what they think about your product or service. What’s not to like about that?