The Danger of AI Model Collapse: When LLMs are Trained on Synthetic Data
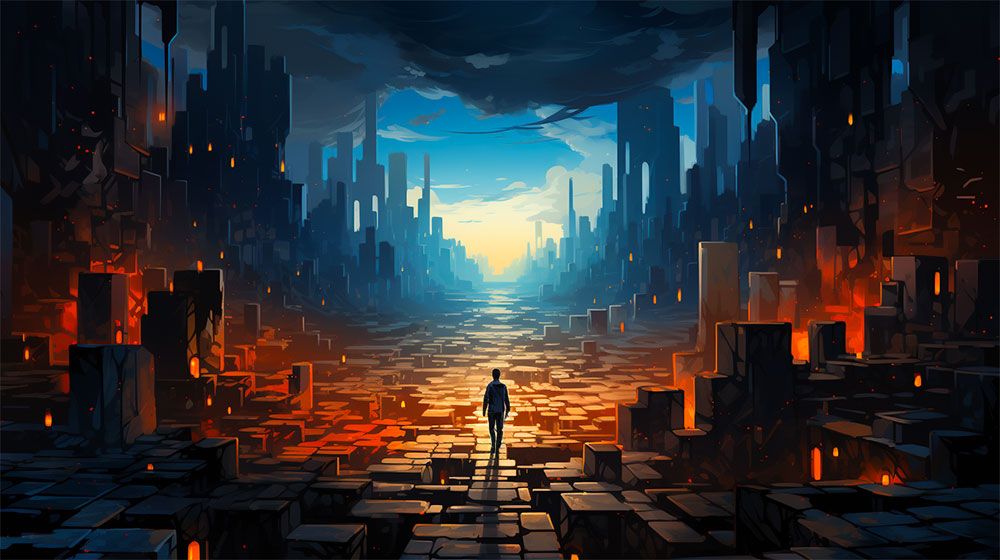
As automation and Artificial Intelligence (AI) technologies advance, there is a growing trend of using AI algorithms to generate written articles, blog posts, product descriptions, images, videos and other types of content. While AI-generated content can provide efficiency, scalability, and democratises creativity (I certainly like to come up with prompts to add some visual stimulation to my posts using Midjourney, although I am certainly not an artist), there is a risk of sacrificing quality and uniqueness.
If organisations solely rely on AI-generated content without human oversight and editing, they may end up with a vast amount of low-quality content that lacks originality and fails to engage readers. Moreover, an overreliance on AI-generated content can also lead to a lack of diversity and creativity in the content produced. Let me explain why.
AI algorithms operate based on patterns and existing data, which means they may replicate common structures and phrases, resulting in a homogenised output. Consequently, readers may encounter a flood of content that appears generic, repetitive and lacks the unique voice and perspective that human creators bring. More importantly, these biases will be amplified if this data is used to train the next round of machine learning models.
This is the topic of this week's article, as I will explore the ethical implications, bias reinforcement, and unforeseen consequences that may arise when AI models are trained on their own content. By shedding light on the ethical challenges and unintended outcomes of this feedback loop, I hope to ignite a vital discussion that guides us toward the responsible development and deployment of AI.
The Role of Synthetic Data in AI Model Training
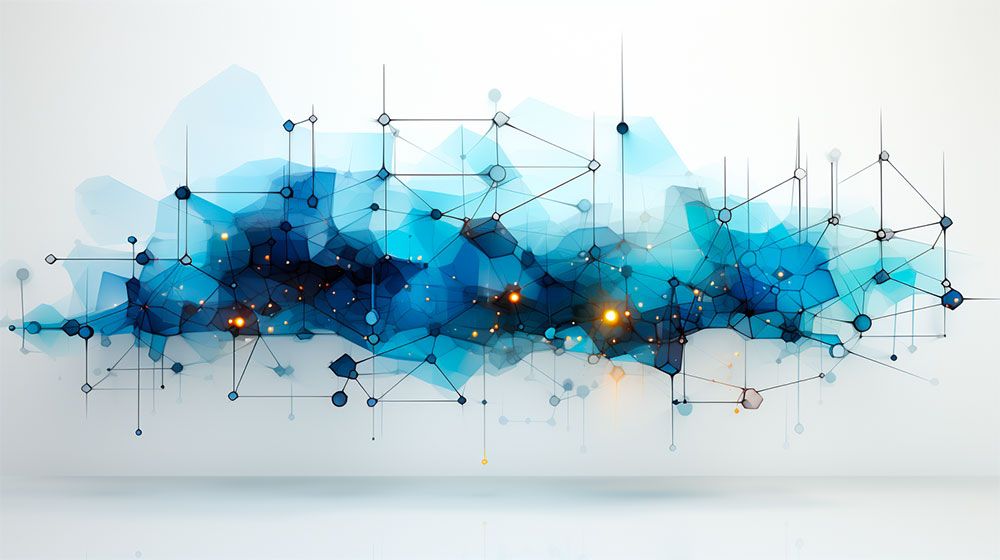
AI models are typically built using machine learning techniques, where they are trained on labelled datasets to learn from examples and generalise their knowledge to new data. The training process involves feeding the AI model labelled data, allowing it to identify patterns and relationships between input data and corresponding outputs, also known as supervised learning.
Through an iterative process known as "training epochs," the model adjusts its internal parameters to minimise errors and improve performance. Training data is typically sourced from external datasets and carefully curated and labelled by human experts to guarantee accuracy and reliability. However, data scarcity is increasingly becoming a challenge, where obtaining a large and diverse labelled dataset is difficult, and researchers turn to synthetic data to overcome this problem.
Benefits of Synthetic Data
Synthetic data addresses this by generating ample labelled data, enhancing the training of AI models. Researchers deliberately use synthetic data when they need to generate artificial data that mimics the characteristics of real data. This approach is beneficial in several scenarios and offers several benefits
Synthetic data is cost-effective, as generating synthetic data can be automated, reducing the need for extensive data collection and labelling efforts.
1. Synthetic data can be used to protect sensitive or private information. Generating artificial data that closely resembles the original data is helpful to researchers so they can conduct experiments and share findings without compromising privacy.
2. Synthetic data enables the creation of highly diverse datasets, covering a broader range of scenarios than the original data, leading to improved generalisation and performance of AI models.
3. Synthetic data enables data augmentation, where synthetic data can supplement real data, exposing models to a wider range of scenarios and improving their ability to generalise.
4. Synthetic data allows for controlled experiments, enabling researchers to manipulate aspects of the data generation process and gain insights into model behaviour and performance.
Challenges of Synthetic Data
However, synthetic data introduces challenges as it perpetuates biased patterns and distributions, resulting in biased AI models even if biases were not explicitly programmed, leading to discriminatory outcomes and reinforcing societal inequalities. The lack of transparency and accountability in synthetic data generation also poses challenges, as understanding how biases and limitations are encoded in the data becomes difficult, hindering efforts to address and rectify biases.
It is important to understand that there is a significant distinction between deliberately using synthetic data and inadvertently utilising it, as exemplified by large language models (LLMs) trained on internet data. Deliberate use of synthetic data involves purposefully generating artificial data that resembles real data, serving specific research or application needs. This approach can be highly valuable, especially when real data is scarce, or privacy concerns arise. However, the unintentional use of synthetic data, such as when LLMs are trained on internet data that increasingly consists of AI-generated content, is a different problem and can make future LLMs increasingly useless if not taken care of this problem.
The Problematic Feedback Loop
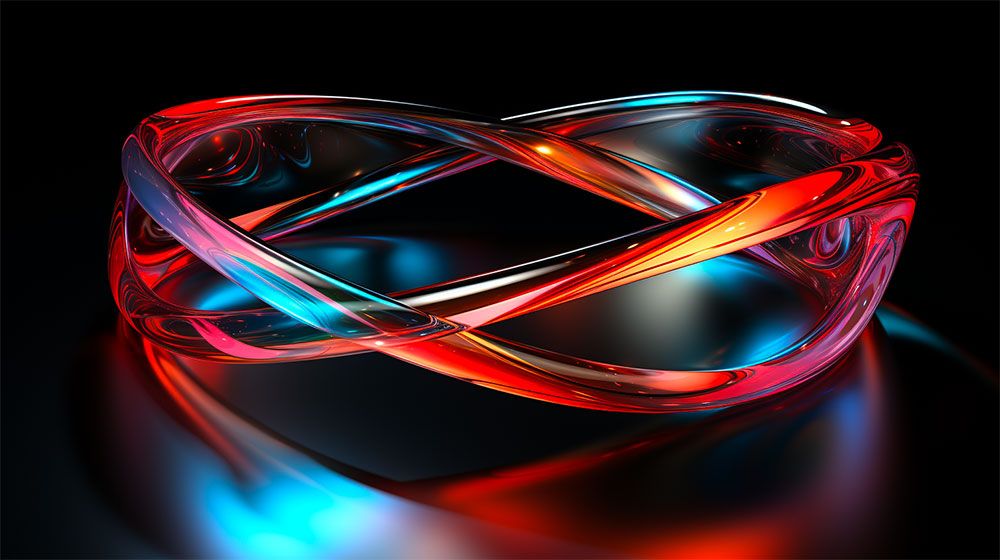
A problematic feedback loop can emerge when AI models are trained on their own content. This loop occurs when the model generates, analyses, and learns from its own data, perpetuating biases and limitations. Without outside assistance, the model's outputs start to reflect its inherent biases more and more, which could result in unfair treatment or skewed results. Recognising and addressing the consequences of this loop is vital for responsible AI development, especially when it comes to LLMs. Researchers are exploring methods like adversarial training and diverse data selection to break the loop and mitigate biases.
An insightful research paper from May 2023 titled "The Curse of Recursion: Training on Generated Data Makes Models Forget" raised this concern regarding training AI models on their own content. The study explores the phenomenon of models forgetting crucial information as they rely more heavily on self-generated data.
Researchers from different universities discovered that when AI models are trained exclusively on their own content, they tend to prioritise recent information over previously learned knowledge. This prioritisation often leads to a phenomenon known as catastrophic forgetting, where the model's performance on previously learned tasks significantly deteriorates.
AI That Forgets
The implications of this curse of recursion are far-reaching. AI systems that forget crucial information can be unreliable, prone to errors, and lack the ability to retain knowledge accumulated over time. This limitation hinders their practical applications in domains where historical context and consistency are vital.
The feedback loop created when AI models are trained on their own content significantly impacts how they perceive reality. This distorted interpretation can perpetuate biases, societal prejudices and fail to capture the complexity of the real world.
The research paper highlights the need for a balanced training approach that combines self-generated data with external datasets to prevent catastrophic forgetting. Models can maintain a more thorough understanding and retain the knowledge acquired from different sources by incorporating a mix of fresh and diverse information during training.
This study is a stark reminder of the complexities and challenges associated with training AI models on their own content. While the ability to generate and learn from self-generated data offers exciting possibilities, it also necessitates careful consideration and implementation of strategies that mitigate the risks of forgetting crucial information.
A growing concern is the unintentional feedback loop resulting from the widespread usage of generative AI tools like ChatGPT or Midjourney. As more individuals turn to these tools to create content, synthetic media becomes an unavoidable part of the training sets. This situation raises a host of concerns about the potential impact and unintended consequences that arise when we heavily rely on synthetic data in AI systems, also known as model collapse.
Model Collapse: Understanding its Implications
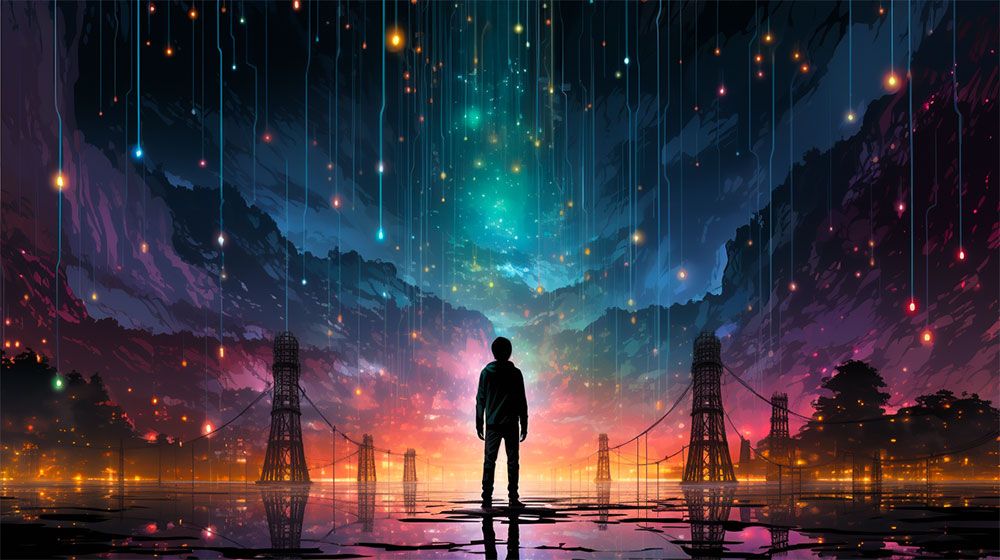
Model collapse is a significant concern that arises when training AI models on their own content. Understanding the concept of model collapse and its implications is crucial for grasping the risks associated with this training approach.
In AI training, model collapse refers to a phenomenon where the AI model fails to generalise properly and produces repetitive or redundant outputs. This collapse occurs when the model becomes overly reliant on a limited subset of its training data, often its own generated content, without effectively capturing the diversity and complexity of the broader dataset. The consequences of model collapse can have far-reaching implications, affecting the reliability, accuracy, and ethical aspects of AI-generated content.
Misinterpretation of Reality
When AI models experience model collapse, they may develop a distorted perception of reality. These models might fall short of accurately capturing the complexities and nuanced aspects of the real world by focusing on a constrained set of inputs. Consequently, their output may not align with objective reality, leading to biased or incomplete understandings.
Potential Biases and Distortions in AI-Generated Content
Model collapse can perpetuate biases and distortions within AI-generated content. If the training data is biased or limited, the model's outputs may exhibit the same biases or amplify existing distortions. This can have adverse effects, reinforcing societal prejudices or producing content that misrepresents certain groups or situations.
Decreased Reliability and Accuracy of AI Models
Model collapse compromises the reliability and accuracy of AI models. The models may become too focused on recurrent or redundant patterns, which could prevent them from generalising or adapting to novel situations. This decreases their reliability in providing accurate predictions, recommendations, or decision-making, potentially leading to erroneous or unreliable outcomes.
It becomes essential to diversify the training data and include outside sources to address the effects of model collapse. AI models can avoid the drawbacks of model collapse and produce more precise, unbiased, and dependable outputs by incorporating a wider range of inputs and ensuring diversity.
Exploring Potential Solutions and Mitigations
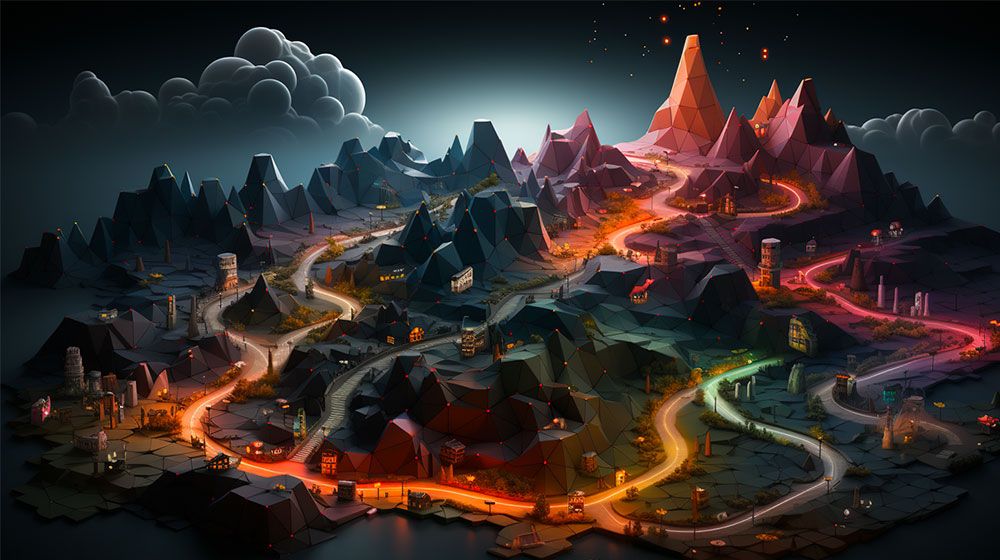
LLMs can indeed generate high-quality content when trained on well-curated and diverse datasets, but there are risks associated with unintentional exposure to synthetic content. For example, the lack of proper curation and verification processes means that the models can learn from inaccurate, misleading, or poorly generated synthetic data. This can result in outputs that lack coherence, relevance, and accuracy of the content produced.
Model collapse shows why addressing these issues is crucial and finding responsible ways to navigate the evolving landscape of AI-generated content. To address the risks associated with training AI models on their own content and mitigate the challenges discussed earlier, there are two options available:
1. Verify Synthetic Content Before Using it as Training Data
A simple solution to ensure that synthetic content does not inadvertently find its way into the training set is by verifying whether a piece of content is AI-generated or human-generated. Finding effective methods to discern and filter out synthetic content is essential to maintain the integrity and reliability of training data in AI systems. Unfortunately, developing robust mechanisms and advanced verification techniques to filter out synthetic content at scale is challenging are critical steps towards addressing this concern and upholding the quality of training datasets.
2. Create Language Models Using Smaller Datasets
When Sam Altman stated that the era of very large language models trained on vast amounts of data is over, he refers to a shift in the direction of AI research and development. The statement suggests recognising the limitations and challenges associated with scaling up language models without carefully considering the potential risks and unintended consequences.
Altman's statement indicates a call for a more thoughtful and responsible approach to AI model development. It suggests a shift towards focusing on improving the quality, interpretability, and reliability of language models rather than solely pursuing larger-scale and more data-intensive models.
To achieve this, alternative training strategies are being explored, such as incorporating diverse and representative training data to reduce the risk of model collapse. Techniques like regularisation, curriculum learning, reinforcement learning, and fine-tuning are being employed to address specific challenges and improve the models' performance.
It is important to note that the effectiveness of these strategies varies based on factors like model architecture, dataset composition, and training objectives. Ongoing research and experimentation are crucial to gain deeper insights into mitigating model collapse and fostering the robustness and diversity of generated content.
Future Considerations and Recommendations for Organisations
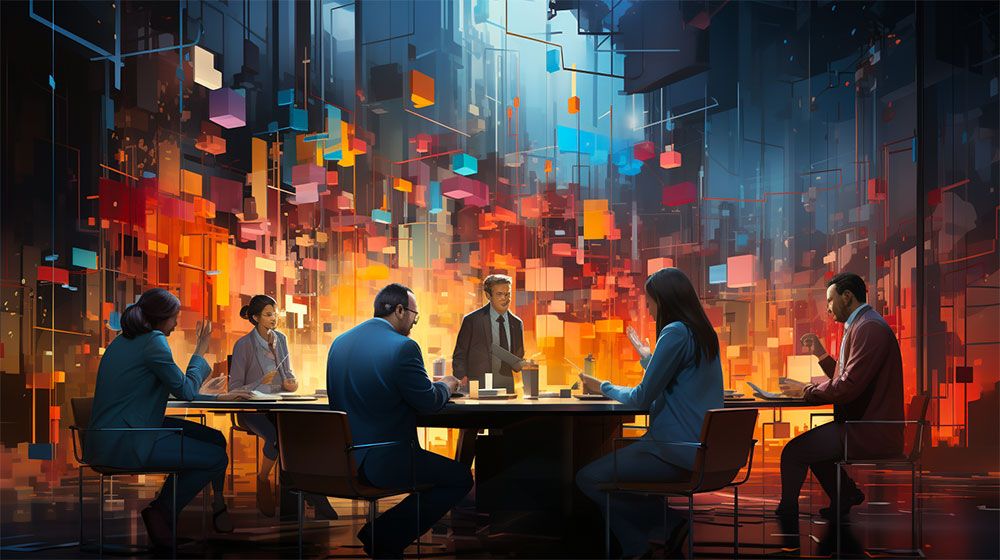
When training AI models using synthetic data (deliberately or unintentionally), several insightful considerations and comprehensive recommendations can guide future practices and ensure responsible AI development. These include the need for further research, collaborative efforts, and responsible AI development practices. Let’s briefly explore these issues.
Need for Further Research
Continued research is crucial to fully comprehend the implications and challenges associated with training AI models on their own content. This research should delve into effective strategies to mitigate biases, enhance transparency, detect synthetic content and ensure the ethical use of AI. If we expand our understanding, we can proactively address potential pitfalls and develop more robust frameworks for AI development.
Collaborative Efforts
Collaboration between researchers, organisations, and policymakers is essential to navigating the complexities of training AI models on ever-larger datasets. These stakeholders can establish standards, define best practices, and create legal frameworks that promote ethical AI development. With a collaborative ecosystem, we can collectively tackle challenges, exchange knowledge, and foster responsible innovation in AI.
Responsible AI Development Practices
Organisations must prioritise fairness, transparency, and accountability in their AI development practices. This involves incorporating diverse perspectives throughout the process, including diverse representation in the teams developing and testing AI models. Clear guidelines for human oversight should be established to ensure that AI models are trained and fine-tuned with appropriate human intervention. Implementing robust bias detection and mitigation mechanisms is crucial to address any biases that may be inadvertently perpetuated through the training process.
Final Thoughts
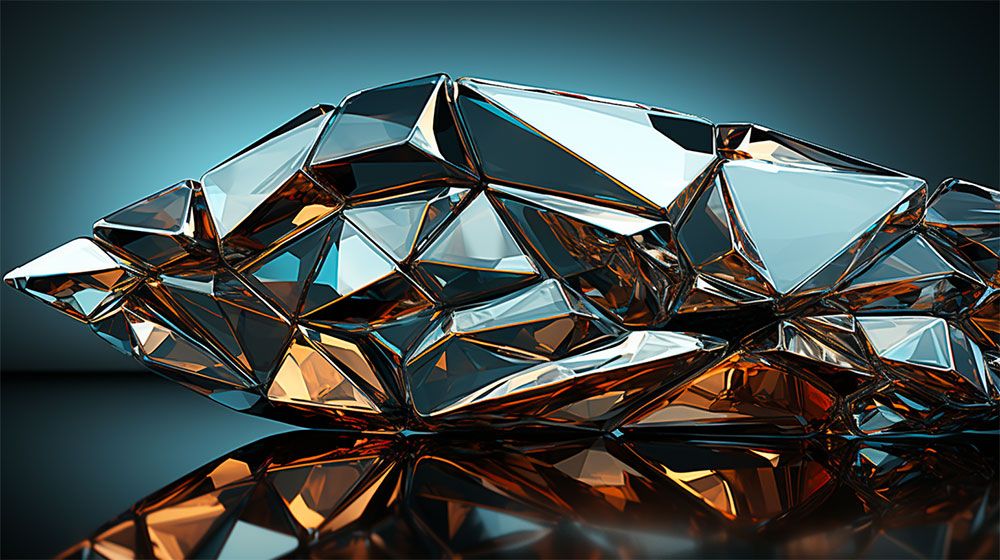
The dangers of AI models trained on their own content highlight the need for caution, responsibility, and ongoing evaluation in AI development. While the capabilities of AI are promising, it is crucial to recognise the limitations and potential risks associated with this training approach.
As AI becomes more integrated into our daily lives and organisational processes, it is essential to prioritise diversity, transparency, and human oversight. We can lessen biases, improve accuracy, and encourage inclusivity in AI-generated content by maintaining human-generated datasets, adding fresh human content, varying the sources of training data, and ensuring ongoing human evaluation.
Collaboration between researchers, organisations, and policymakers is paramount. We can tackle the difficulties and moral ramifications of training AI models on their own content by cooperating, exchanging knowledge, and developing responsible AI practices. This collaborative approach will pave the way for a more responsible and accountable AI landscape.
Let’s move forward with a shared commitment to responsible AI development, ensuring that AI technologies are used for the betterment of humanity, respecting diversity, and upholding the values we hold dear. We can work together to create a future where AI improves our lives while upholding fairness, transparency, and ethical decision-making.